Team : SIBARITA
Research Projects
Combining deep-learning with geometric and image processing for analysis of microscopy data
Project Leader(s): Florian Levet
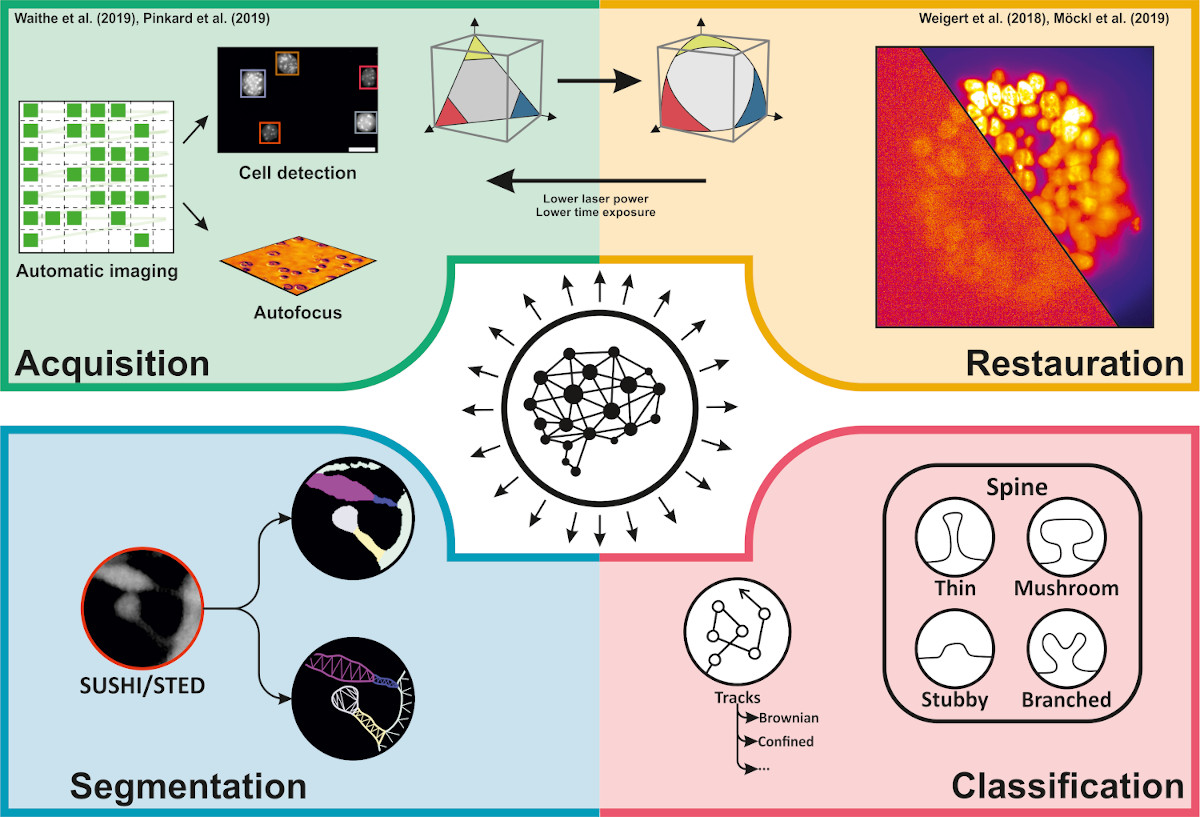
Collaborators: V. Viasnoff (MBI, NUS), M. Nikolski (Labri), O. Thoumine (IINS).
Optical microscopy is an indispensable tool for medical and biological research. Even with the recent advent of super-resolution microscopy, it remains limited by the optics of the microscope, the chemistry of the fluorophores, and the maximum photon exposure tolerated by the sample, necessitating trade-offs between imaging speed, spatial resolution, light exposure, and imaging depth. Deep-learning (DL) has become a very popular artificial-intelligence (AI) method providing a powerful tool for surveying and classifying biological data. Deep-learning algorithms take raw features from a large, annotated data set, such as a collection of images or genomes, and use them to create a predictive tool based on patterns buried inside. A series of high impact articles have recently demonstrated that deep learning algorithms are extremely useful to accelerate image acquisition and improve image quality in fluorescence microscopy, extending the range of biological phenomena observable by microscopy.
Relying on the recent developments achieved in the team, our goal is to significantly improve bioimage acquisition and analysis workflow performances by i) accelerating the image acquisition, ii) reducing the photo-toxicity, iii) optimizing important acquisition parameters, and iv) segmenting and classifying biological structures of interest. In this context, deep-learning will be used to automatically restore, classify and segment images. We will also use our experience in geometric and image processing to facilitate image annotation for training, as well as improve the analysis throughput of the deep-learning approaches. This work aims at paving the way toward a so-called “smart microscope”: an “intelligent” microscope offering advanced acquisition and analysis capabilities, beyond physics.
Fundings
None